
- This event has passed.
Machine learning in plant–pathogen interactions: empowering biological predictions from field scale to genome scale
07/12/2022 @ 09:00 - 10:00
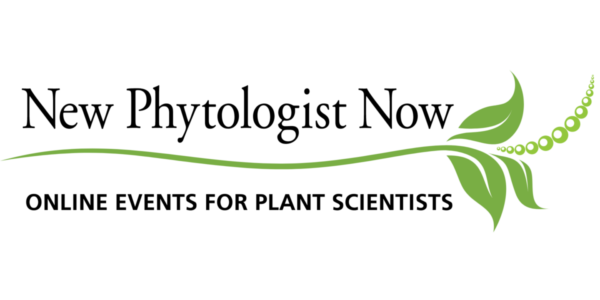
Machine learning (ML) encompasses statistical methods that learn to identify patterns in complex datasets. Here, Jana reviews application areas in plant–pathogen interactions that have recently benefited from ML. She provides an under-the-hood glance into her developed suite of ML-based tools for pathogen effector prediction such as EffectorP. Jana will discuss common pitfalls and challenges she encountered during the development of ML approaches. Finally, she will highlight future opportunities for ML as a tool for dissecting plant–pathogen interactions, for example through integration of AlphaFold predictions or ML-driven effector gene annotation.
——
Jana is a bioinformatician interested in the interactions between plants and their pathogens. Her work involves using computational techniques to gain new insights into plant–pathogen relationships, and her contribution includes widely used software tools for fungal effector prediction using machine learning. She completed a M.Sc. in Computer Science with a focus on Bioinformatics at the University of Freiburg, Germany. In 2008, she commenced a Ph.D. at the University of Western Australia in which she developed novel methods for the computational prediction of non-coding RNA structure. In 2012, she joined CSIRO as a Postdoctoral Fellow to work on plant-pathogen interactions. In 2018, she joined the Australian National University as a Research Fellow at the Biological Data Science Institute and was awarded a 2019 ARC Discovery Early Career Researcher Award (DECRA) to uncover how rust fungi cause devastating plant diseases. In 2021 she joined CSIRO as a Senior Research Scientist where she leads the Data Intensive Approaches team. Jana’s Tansley insight ‘Machine learning in plant–pathogen interactions: empowering biological predictions from field scale to genome scale’, focuses on machine learning (ML) applications in areas in plant–pathogen interactions and highlights benefits and challenges in using ML approaches.